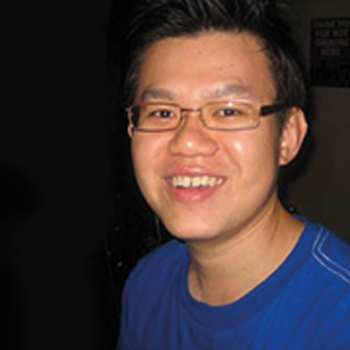
How machine learning is applied in industrial IoT
Unlike data-driven models that may contain biases, industrial machine learning is grounded in the laws of physics. “We’ve been able to build physical models to capture our understanding of devices and simulate things that haven’t happened yet in the real world,” Bloom said.
However, Bloom conceded that there are some drawbacks in industrial machine learning. For one, physical models do not easily learn from new data, and not all physical laws have been built into large complex systems.
“That means we have an imperfect model, and we just hope that the imperfections are not fatal,” he said, adding that refinements to industrial machine learning models are not easy to make either, since experts with doctoral degrees are often needed in specialised fields such as metallurgy.
A data-driven model, however, does not require explicit understanding of the physical world, Bloom said. “You just throw in lots of data and optimise the model based on metrics that you’ve set up. It will naturally improve as you throw more data at it, and it’s extensible to more data sources.”
That said, Bloom noted that there is uncertainty over whether data-driven models can be generalised to other similar systems. “It’s also difficult to derive real physical intuition for those models,” he added.
At GE, work is underway to combine physical and data-driven machine learning models in its systems, and to ensure that the information generated from those models is trusted and understood by users. “Even if the models are correct, if people don’t trust and accept them, they are not going to wind up being used,” Bloom said.
“In the end, we need to create not just better algorithms, but also make machine learning suggestions understood to people with domain expertise. We also need to build systems that take in feedback, and are cognizant of the end user and the effects of a good and bad answer.”