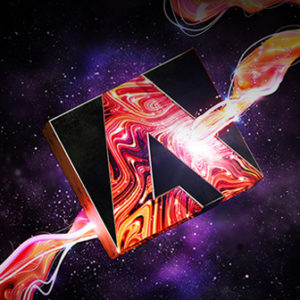
Getting Machines to Think: A Primer on Artificial Intelligence
The world has seen an explosion of technology, from the internet to mobile, but now artificial intelligence (AI) is ushering in the next wave of innovation.
AI, the process of getting computers to think more intuitively, is making amazing experiences become so commonplace that they blend into the fabric of everyday life.
AI is working when your smartphone recommends an Italian restaurant that has the best pasta primavera you’ve ever tasted. It’s there when your bank sends you a text asking if you just charged $2,000 on your credit card to a bridal shop that’s 3,000 miles away. And when your photo of a tropical beach is marred by a beach-reveler, AI can make him disappear.
By 2020, businesses that use AI and related technologies like machine learning and deep learning to uncover new business insights will take $1.2 trillion each year from competitors that don’t employ these technologies.
“There are all sorts of ways business is done today that will be disrupted and transformed by AI, from how medical advice is dispensed to how marketing campaigns are developed,” says Gavin Miller, head of Adobe Research. “It’s game-changing.
AI promises to create transformative experiences across several industries, and creatives, marketers, businesses, and their customers will all benefit from these advances. However, for even the most technical people, AI and its associated terminology can be confusing. Understanding AI’s various parts is key to understanding the power of this technology, so here’s primer for everything you need to know about AI and the capabilities it offers that will drive digital transformation in the coming years.
AI from A to Z
Artificial intelligence is steeped in daunting terms, such as “machine learning” and “neural networks.” By looking at the evolution of AI, you can see how the different elements of AI fit together, and how AI is helping creatives, marketers, and others do their work more effectively.
All about AI
From the time people began telling stories, they have imagined artificial intelligence, such as “the golden robots,” found in ancient Greek myths, that could think independently and serve the gods. The science of artificial intelligence first emerged in the ‘40s and ‘50s, when academic scientists began pondering the notion of an artificial brain. Theorist Alan Turing proposed the so-called “Turing Test” in 1951 to assess whether a computer could think. He is credited with shaping what we know today as artificial intelligence. However, AI’s modern usage traces back to the 1980s when AI was applied to “expert systems” that allowed computers to reason by following a series of “if-then” rules — i.e., instructions to the computer that if this happens, then do that.
Much of AI’s work is about analyzing data and making predictions. Consider what happens when you go to an online market. AI can analyze vast amounts of information and instantly offer up suggestions for products you might want to buy based on how you are zipping around online, your past buying habits, and the buying habits of other people like you.
Increasingly, AI is also an element in the creative field. When you search for the perfect stock image, AI is at work matching your search criteria to the best image for what you need.
Making sense of machine learning
Traditionally, AI was based on human computer programmers developing algorithms — step-by-step instructions for how computers should do tasks. Computers couldn’t do anything unless they were explicitly directed to by the programmer, which is a time consuming task. In a sense, machine learning unshackles computers. As the computer is exposed to more and more data, it can see connections in the information that allow it to develop more precise predictive models without needing explicit instructions from the programmer. The models are continually tweaked as more data comes in, providing better predictions.
A great example of machine learning at work is in Photoshop. Imagine you are editing a photo of a city skyline and you want to remove an airplane in the sky. Photoshop, using machine learning to understand the image data, can enable you to select the airplane, remove it, and seamlessly fill in the space with perfectly-matching sky.
Knowing the neural network
Just as there are subsets of AI, there are different kinds of machine learning. One example is artificial neural networks — a way of processing information that was inspired by the way the human brain works. The brain uses a large number of neurons working in unison to solve complex problems. An artificial neural network takes a similar approach, solving problems by using a large number of interconnected processing elements.
While this is admittedly a highly complex process, Jon Brandt, director at Adobe Research, says the underlying principle is fairly simple in terms of the computer learning from data to find better solutions. He gives the example of a data model that identifies faces in photographs so they can be automatically tagged.
A computer programmer developing such a model might assume the best way to recognize a face in a photo is to measure the distance between the eyes and other facial features. Inherently, the resulting data model might revolve around that assumption. But what if there were a better method the programmer never considered?
Because neural networks and machine learning aren’t restricted by the programmer’s explicit instructions, they can analyze data in a different way and come up with insights that aren’t obvious or expected. The neural network can find correlations in data that the human programmer never imagined — resulting in better ways to tag photos.
While the subtleties of the different AI, machine learning, and neural network approaches can be difficult to grasp if you aren’t a computer programmer, it’s important to remember that AI and machine learning are simply ways of doing mathematical calculations.
Each step in the evolution of AI shares a similar characteristic: it provides better answers and doesn’t require computer programmers and end users to understand what’s going on under the hood or to do the repetitive tasks that are necessary to generate the best results.
Preparing AI for the future
AI and machine learning has matured thanks to a combination of more robust computing power and available data — and this will help many companies effectively apply it to the needs of their business. AI capabilities can reduce the amount of time spent on repetitive, time-consuming tasks.
Still, even though AI and machine learning are being used today for many important purposes — from fraud detection to product recommendations — the technology is still in its early stages. And while the promise of AI is tantalizing, there are still limitations and issues that need to be addressed before that promise is fully realized.
For example, Jon says that we have become so accustomed to asking our phones a question and receiving an answer that we take the act of speaking to a computer for granted. In reality, the current interactions are relatively simple. Having a computer understand verbal directions about how you want a photograph altered, and then handling that task with the skill of a human assistant is much more daunting. But that is the ultimate goal of AI: to liberate creatives, marketers, business leaders, and others from the tedious aspects of using technology.
In addition to the technical challenges involved with AI, the societal implications of the greater use of AI are being widely debated. Since AI is fueled by using more data and different kinds of data, we must be sure that the data is protected and used in responsible ways.
On a personal level, people are curious about what will happen to their jobs when AI reaches its full potential. Jon understands that concern, but he believes AI will not replace people so much as handle some of the menial tasks they must do, so they can concentrate on more important things.
“When a new technology is introduced, like cameras, digital imaging or even the printing press, the historic pattern has been for the technology to become yet another paintbrush for human creative expression,” Jon says.
The question, then, is what is required for AI to reach its full potential. Jon says it’ll take a multidisciplinary-spanning, collaborative vision, language specialists, cognitive scientists, human-computer interaction, and the creatives themselves to address this challenge.
“Part of what I am describing is interdisciplinary,” Jon says. “It takes a diverse set of skills and people coming together (and part of that is really solving the language problem), which is how we can robustly understand both the intent and the content of the message.”
Still, Jon sees AI becoming an integral part of the way we work, live and create.
“Ultimately, you can think of AI as being the pinnacle of human-computer interaction, in that it mediates all of our interactions with machines,” he says.
Read more stories from our artificial intelligence series by visiting this page. For more information about Adobe Sensei, visit http://www.adobe.com/sensei.html