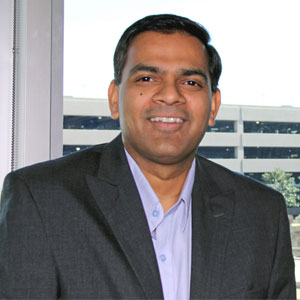
6 steps to create value from Machine Learning for your business
A thousand years from now when someone writes the history of the human race, the emergence of Machine Learning will be hailed as a significant milestone. Machine Learning (ML), a branch of Artificial Intelligence (AI), enables computers to learn from data without being explicitly programmed. Today, ML has established itself as the key to unlocking the value from customer data. Netflix’s movie recommendations, Facebook’s ability to spot our faces, Google’s self-driving cars are all early examples of ML-powered solutions. However, ML and AI are still in a nascent stage with the majority of industry leaders still struggling to cut through the hype and set right priorities for their businesses. Given the largely untapped potential of this innovation, the following steps can serve as a strategic roadmap for building ML capabilities that help create tangible value for your business.
Step I: Build data fabric for your organization
Despite living in a data-driven economy, we do not give data the respect that it deserves. The only time data is considered an asset is when a catastrophic data breach occurs; then it becomes our most valuable possession! Creation of data fabric, an ecosystem that would provide seamless well-governed data integration across the enterprise, is one of the most critical steps for deploying ML solutions. Without an efficient and well-designed data fabric, valuable data will be stuck in silos and never get organized, democratized, and monetized to the fullest extent. Without this foundation, ML would become a ‘garbage-in garbage-out’ exercise leading to dangerously inaccurate decision-making with detrimental customer experience.
“L and AI are still in a nascent stage with the majority of industry leaders still struggling to cut through the hype and set right priorities for their businesses”
Step II: Hire the right talent
A common ML human capital strategy is to hire ‘unicorn’ data scientists with doctoral degrees, exceptional computer programming skills, extraordinary analytical prowess, and brilliant business acumen. In reality, it is simply impossible to find these mythical creatures! They are called unicorns because they are truly figments of our imagination. At its core, ML is a team sport and a cross-functional team comprising of a domain expert, a statistician, and a data engineer can accomplish much more than three unicorn data scientists put together. Another common occurrence is favoring only one particular algorithm such as xgboost (extreme gradient boosting machine algorithm). It is important to hire data scientists who regard these algorithms as tools in the toolkit rather than magic potions to solve any problem instantly. Bringing right external talent onboard and creating internal talent pipelines are essential prerequisites for the successful evangelization of ML across the enterprise.
Step III: Create a lab environment
Machine Learning is a scientific field and science happens in a laboratory. Successful and rapid prototyping requires a lab environment with access to all enterprise data assets, state-of-the-art analytic tools, and the ability to run champion-challenger tests. With such a set-up, the cross-functional team can quickly move from defining the business problem to conducting analytic experiments, and ultimately developing a ‘minimum-lovable’ challenger ML model with greater prediction power or deeper consumer insights. A lab environment allows the team to undertake a large number of research initiatives, recover quickly from failed experiments, and ultimately identify the most valuable ML applications for the business.
Step IV: Operationalize successful pilots
Once the successful pilots are identified, the next logical step is to deploy them in customer-facing business strategies and operations. This is where the rubber hits the road and unfortunately melts very quickly! Using inappropriate technology infrastructure, bad software engineering practices, and lack of model governance turns even a great model into an absolute disaster. The emergence of container platforms has enabled packaging ML tasks into standardized units for development, shipment, and deployment. However, this requires orchestration across different operating systems while maintaining the highest performance standards. This is why the projected demand for data engineers will likely to be much higher than that for data scientists in the near future. Nevertheless, successful deployment and operationalization of few critical ML models will build the necessary momentum for wider deployment across the organization.
Step V: Scale up for enterprise-wide adoption
Value creation within an organization takes different forms but at the end of the day, it is all about making key business processes better, faster, or cheaper. Every business executive should conduct a comprehensive audit of their key processes and identify potential opportunities for supervised and unsupervised ML algorithms. Once a list of potential applications identified, new initiatives should be prioritized based on the basis of incremental business value and potential customer benefits. However, there are several industry-specific constraints that play a big role in determining the choice of ML algorithm. For instance, consumer finance regulations set a very high threshold on model interpretability; hence the banks need to be very careful before applying black-box methodologies such as artificial neural networks. However, this is an active research area and several open-source projects such as LIME (Locally Interpretable Model-agnostic Explanations) are gaining a lot of traction in the industry. Overall it is critical to apply ML within the appropriate business context. As Dr. George Box, one of the greatest statisticians, once said, “Essentially, all models are wrong, but some are useful.”
Step VI: Drive cultural change
Finally, we need an organizational culture that embraces a fundamental shift from classical statistical methods to modern ML methodologies. This transformation is fostered by a ‘continuous learning’ culture where the team is encouraged to embrace their inner ‘geek’ and keep updating their skill-set by learning new programming languages such as R and Python, and open-source Big Data framework such as Apache Hadoop. Furthermore, executive leadership teams should promote a data-driven decision-making culture via healthy dissemination of data and knowledge across the enterprise.
In conclusion, we are witnessing a massive tectonic shift in the business landscape with the emergence and operationalization of Machine Learning for creating more predictive models, deeper consumer insights, and better customer experience. Creation and execution of an ML strategic roadmap is the key to unlock significant business value and help develop long-term competitive advantage for your business.